At this time, purchasing EASY Bot items is not available to all members. Read more - how to get access to purchase
APL Forecast
Understanding APL Forecast
- The APL Forecast methodology revolves around predicting future market behavior using historical price data.
- The model integrates various algorithms to evaluate market dynamics and extract relevant trends.
- Key techniques employed include the Caterpillar-SSA method, which filters out noise and identifies influential factors in price changes.
Algorithmic Components
- Forecast Algorithm: This includes options for vector and recurrent models that can adapt to different market scenarios.
- Data Fragment N: The length of the analyzed price series plays a crucial role in developing a reliable forecast.
- Time-Dependent Lag: This parameter specifies how historical data influences future price predictions, typically set to fractions of N.
Noise Filtering Techniques
- High Frequencies Filtering: The tool allows adjustment of a parameter to suppress noise from high-frequency oscillations.
- Smooth Trend Extraction: Users can manage indicator parameters to smooth extracted trends and control noise filtering thresholds.
Model Quality and Forecasting
- Adequate Model Selection: Emphasizes the importance of selecting statistical models that produce quality forecasts rather than high quantities of them.
- Prediction Points: The system predicts future price values, with a focus on 10 to 30 points for reliable forecasting.
- Implementation Success: Experience and user feedback indicate that the use of APL Forecast with the right parameters can significantly enhance trading strategies. 💹
Strategies for Optimization
- Length of Price Series: It's recommended to use datasets between 200 to 600 price points to boost prediction accuracy.
- Timeframe Selection: Utilizing a coarser timeframe for model analysis may yield better accuracy by observing long-term trends.
- Multiple Indicator Applications: Combining indicators with different parameters can reveal divergences in influence factors across time scales. 🔍
User Insights and Experiences
- Users report that adjusting key parameters during real-time trading have enhanced their forecast accuracy.
- Despite its complexity, positive user reviews affirm that following recommendations can lead to fruitful trading outcomes.
- Community feedback suggests developing mastery over the input variables yields optimal results when employing the APL forecast. 😃
Symbol | Price | Today Forecast | Week Forecast | Month Forecast | Year Forecast |
---|---|---|---|---|---|
A APLM
APLM
|
0.1300
5.69% |
Improve your Trading
Subscribe Telegram
Learn the secrets of successful trading: Get favorable offers for automatic trading algorithms and increase your chances in the market!
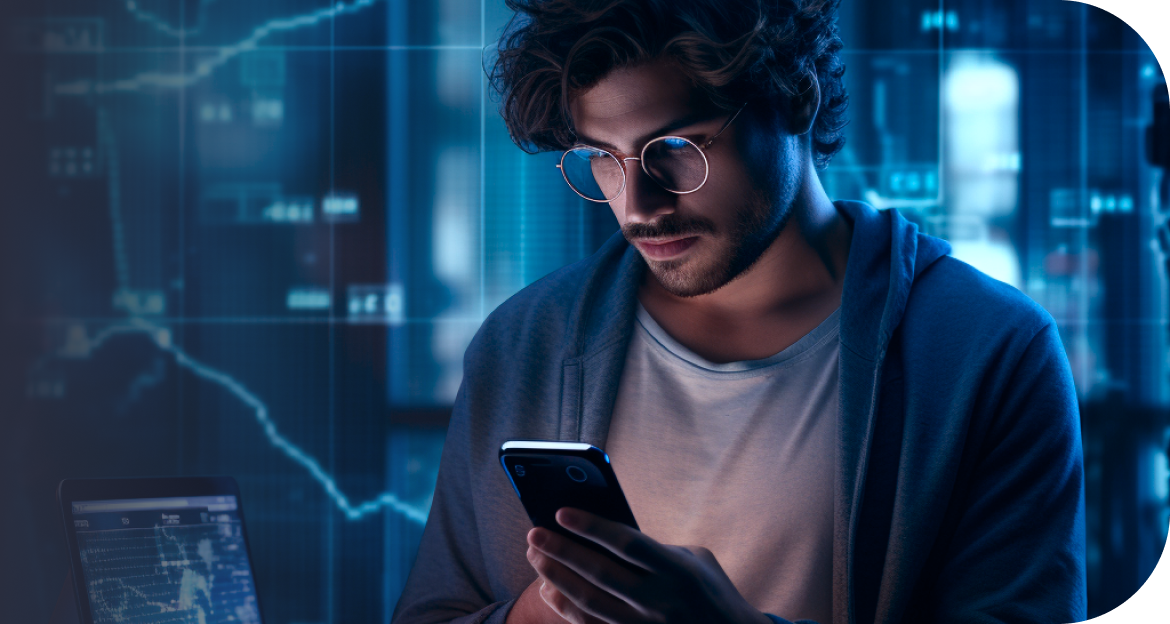